What Are The Key Components Of Data Science?
4 out of 5 based on 12 votesLast updated on 2nd Apr 2025 2.4K Views
- Bookmark
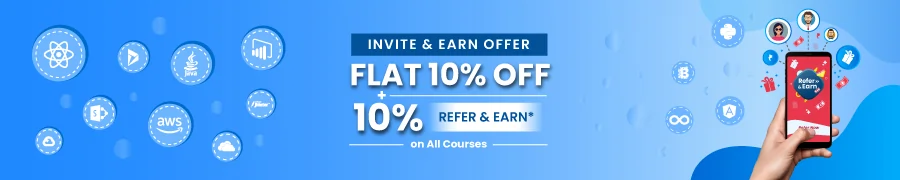
Discover the key components of Data Science, including data collection, preprocessing, analysis, machine learning, and visualization for actionable insights.
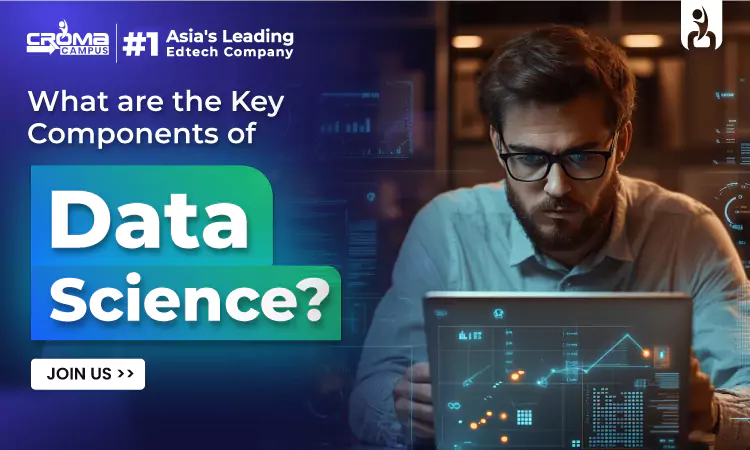
Hyderabad is quickly becoming a leader in artificial intelligence and data analytics. Many tech companies, startups, and research institutes are investing in AI and big data. This has increased the demand for skilled data scientists. If you want to enter this field, joining a Data Science Course in Hyderabad can help you build the right skills.
Data science is a multifaceted field focused on the systematic collection, rigorous analysis, and practical application of data to address real-world challenges. It merges aspects of computer science, statistics, and business acumen to create a comprehensive approach to problem-solving.
While numerous online articles provide an overview of data science concepts, very few delve deeply into the fundamental elements that drive the discipline's success. In this blog, we will thoroughly explore the key components of data science, uncovering their significance and their impact on deriving insights and making informed decisions.
Data Engineering – Preparing the Data
Data engineering serves as the foundational pillar of data science, playing a crucial role in the journey from raw data to insightful analysis. Without meticulously curated and structured data, no analytical process or predictive model can function effectively.
Data engineers are the professionals who ensure that various types of raw data are properly gathered, stored, and transformed into a usable format. Their responsibilities include:
- Data Collection: They pull data from a multitude of sources, which may include relational databases, websites, and application programming interfaces (APIs), ensuring a diverse array of information is captured.
- Data Storage: Once the data is collected, data engineers organize it in systematic repositories such as cloud storage solutions and data warehouses, facilitating easy access and management.
- Data Cleaning and Transformation: They then engage in the essential tasks of cleaning and transforming the data. This involves removing inaccuracies, handling missing values, and reshaping data structures, all aimed at making the data suitable for deep analysis and robust modeling.
Through these careful processes, data engineers lay the groundwork that allows data scientists to derive meaningful insights and drive informed decision-making.
For example, in Ahmedabad, businesses are using AI to improve supply chain management. This requires well-structured data to track goods and predict demand. Companies are looking for professionals with expertise in data handling, and many are enrolling in a Data Science Course in Ahmedabad to learn these skills.
Relevant Data Science Online Courses:
Full Stack Data Science Course
Python Course for Data Science
Machine Learning Online Classes
Azure Machine Learning Certification
Statistical Analysis – Understanding Data
Statistics serves as the crucial groundwork for the field of data science, offering tools and methodologies for interpreting complex datasets. By applying statistical principles, data scientists can uncover trends, identify patterns, and explore relationships within data. Prior to constructing predictive models, these professionals engage in a process known as exploratory data analysis (EDA). This vital step enables them to assess the data's intrinsic properties and glean valuable insights that guide subsequent analyses.
Several fundamental statistical concepts are integral to the practice of data science:
- Descriptive Statistics: This encompasses measures such as mean, median, and standard deviation, which are employed to succinctly summarize and describe the characteristics of a dataset. These statistics provide a quick snapshot of the data's central tendencies and variability.
- Inferential Statistics: This branch involves techniques such as hypothesis testing and probability. It allows data scientists to make informed predictions and inferences about a larger population based on a sample, thus facilitating decision-making processes under uncertainty.
- Feature Engineering: This innovative practice involves the creation of new data features from the existing dataset, enhancing the predictive power of models. By transforming and combining raw data effectively, data scientists can uncover new variables that improve the accuracy and performance of their analyses.
Together, these concepts form the backbone of effective data-driven decision-making, enabling professionals to harness the full potential of the data at their disposal.
In industries like e-commerce and finance, statistical analysis is used to detect fraud, optimize pricing, and understand customer behavior. Many companies offer a Data Science Course in Indore that focuses on statistics for risk analysis.
Machine Learning – Making Predictions
Machine learning allows computers to learn from data and make predictions. It is one of the most important areas of data science. There are different types of machine learning:
- Supervised learning – The computer learns from labeled data (e.g., predicting house prices based on past sales).
- Unsupervised learning – The computer finds patterns in data without labels (e.g., grouping customers by shopping habits).
- Deep learning – A more advanced form of machine learning that uses neural networks (e.g., facial recognition and speech translation).
Many learners are joining a Data Science Course with Placement to gain hands-on experience in machine learning. For example, healthcare companies use deep learning to analyze medical images and detect diseases.
Model Deployment and MLOps – Using AI in Real Life
Building a model is not enough. It needs to be deployed so that people can use it in real-world applications. MLOps (Machine Learning Operations) helps manage AI models after they are created.
This involves:
- Model deployment – Making models available through web applications or cloud platforms.
- Continuous monitoring – Checking if the model is still accurate over time.
- Retraining models – Updating models with new data to keep them relevant.
Banks and cybersecurity firms use AI models to detect fraud in real-time. To work in these industries, many professionals join a Full Stack Data Science Course to learn how to deploy and maintain AI models.
You May Also Read:
Python Interview Questions and Answers
Data Science Interview Questions and Answers
Table: Key Components of Data Science
Component | Purpose | Tools Used |
Data Engineering | Collect and prepare data | SQL, Apache Spark |
Statistical Analysis | Understand data patterns | Pandas, NumPy |
Machine Learning | Make predictions using data | Scikit-learn, TensorFlow |
MLOps | Deploy and maintain AI models | Docker, Kubernetes |
Key Takeaways
- Data engineering ensures that data is collected and stored properly.
- Statistics helps in analyzing and understanding data.
- Machine learning allows computers to predict outcomes.
- MLOps makes AI models usable in real-world applications.
- A Data Science Course with Placement can help professionals gain industry-relevant skills.
Sum up:
Data science is a fast-growing field, and companies are investing in skilled professionals. Whether in Hyderabad’s AI startups or Ahmedabad’s growing analytics industry, learning these key components can open up exciting career opportunities.
Subscribe For Free Demo
Free Demo for Corporate & Online Trainings.
Your email address will not be published. Required fields are marked *
Course Features





