Statistics For Data Science And Business Analysis
4.9 out of 5 based on 2345 votesLast updated on 1st Aug 2024 24.4K Views
- Bookmark
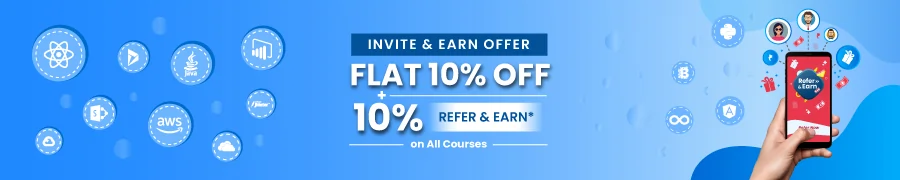
Master Statistics for Data Science & Business Analysis. Learn key concepts, tools, & techniques for data-driven decisions. Enhance your skills with practical applications.
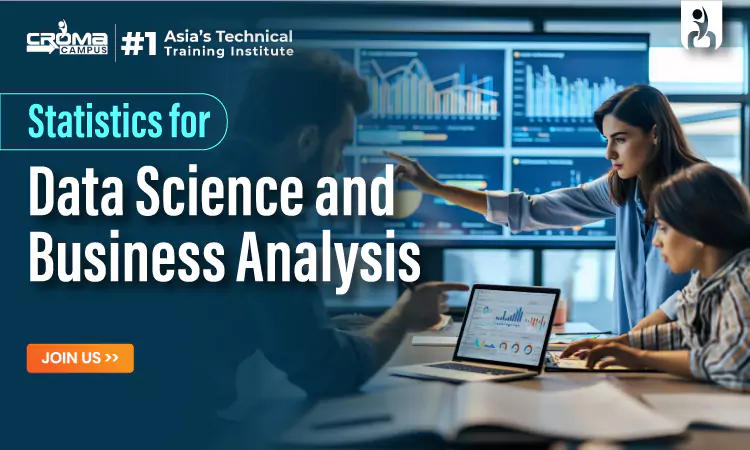
Statistics
is the cornerstone of data science and business analysis in the ever-changing
field of data-driven decision-making. Using statistical tools, professionals
can extract important knowledge from the abundance of information available
today, from finding patterns in large datasets to drawing actionable insights.
We examine the importance of Statistics for Data Science and Business Analysis,
important methods, and practical applications in data science and business
analysis in this blog article.
Introduction to Data Science
Data science
has emerged as a transformational force in this data-driven society. They are
redefining industries and organizational structures. To glean insightful
information from large and intricate datasets. They get insights about data
science that fundamentally integrates statistical analysis and machine
learning.
Data
scientists use sophisticated algorithms and methodologies. They find trends and
forecast outcomes. They also extract useful information that spurs creativity
and helps in decision-making. The uses of data science are numerous and
extensive. This ranges from forecasting consumer behaviour to streamlining
corporate procedures.
In this Data Science Course, we will examine the fundamentals, methods, and
practical uses of data science in this introduction blog post, which will
highlight the field's importance in the current digital era.
Understanding the Significance of Statistics
With
statistics' framework for data analysis and interpretation, practitioners can
make well-informed, empirically supported decisions. Statistics is the
cornerstone of data science, enabling activities like grouping, regression
analysis, and hypothesis testing. In business analysis, statistical methods
help with risk assessment, performance appraisal, and market research.
Key Statistical Techniques
Descriptive
statistics: It sum up and describe a dataset's fundamental characteristics.
Metrics like the mean, median, mode, and standard deviation can infer the
central tendency, dispersion, and form of the data distribution. These methods
offer a preliminary comprehension of the data before further investigation.
Inferential
Statistics: Using a sample of data entails drawing conclusions or predictions
about the population. Confidence intervals and hypothesis testing are two
methods. These are used to measure the uncertainty surrounding these
predictions and evaluate their dependability. Judgments and decisions made by
data scientists and analysts can be made with a certain degree of confidence.
Regression
Analysis: Analyzing relationships between different independent variables. A
dependent variable is the purpose of regression analysis. Predictions and trend
analysis are made possible by their assistance in understanding the
relationship between changes in one variable and changes in another. The often-used
regression techniques are polynomial, logistic, and linear regression.
Probability
Distributions: In a random experiment, probability distributions simulate the
possibility of various outcomes. Understanding distributions, including the
Poisson, binomial, and normal distributions, is essential for various
statistical investigations. These distributions shed light on the likelihood
that certain events will take place in a particular setting.
Data
visualisation: Data visualisation is an essential component of data science and
business analysis. Even if it is not technically a statistical technique.
Visualization tools, including:
- Box plots
- Scatter plots
- Histograms
This makes
it easier for stakeholders to understand insights and trends by visually
intuitively communicating complex statistical data.
Real-World Applications
Marketing
Campaign Optimization: Companies assess a marketing campaign's efficacy through
statistical analysis. By examining consumer reaction data, they can discern
patterns, divide their clientele, and enhance their marketing tactics to
enhance interaction and boost conversion rates.
Financial
Risk Management: Few of the hazards that are assessed and managed in the
financial industry using statistical models:
- Credit risk
- Market risk
- Operational risk
Financial
institutions can minimise risks and optimise profits by making well-informed
judgments based on historical data and market trends analysis.
Healthcare Analytics: Medical professionals use statistics to evaluate patient information and identify illnesses. Regression and survival analyses are two statistical methods useful for determining risk factors, creating treatment programs, and enhancing patient care.
You May Also Read:
Python Programming for Beginners
Python Interview Questions and
Answers
Data Science Interview Questions and
Answers
What is Business Analysis?
The
discipline of recognizing needs and coming up with solutions for business
issues is known as business analysis. It entails assessing its systems and
procedures to increase productivity, profitability, and efficiency within a
business. To guarantee that technological solutions are in line with company
goals.
Understanding
an organisation's current situation, pointing out development opportunities,
and suggesting solutions to meet those needs are the main goals of business
analysis. This frequently includes interviewing stakeholders, compiling
requirements for new systems or procedures, and collecting and evaluating data.
To make sure that everyone is working toward the same objectives, business
analysts also help departments within an organization communicate and
collaborate with one another.
Conclusion
In summary,
statistics is a fundamental component of data science and business analysis. It
enables experts to glean insightful information from data and support
well-informed decision-making. Using an extensive array of statistical methods,
professionals can decipher intricate patterns and forecast forthcoming trends.
Statistics opens up fresh avenues for advancement and creativity. The Data Science course in Delhi will play an increasingly important role in
determining the direction of data driven businesses, and the complexity of data
continues to expand.
Subscribe For Free Demo
Free Demo for Corporate & Online Trainings.
Your email address will not be published. Required fields are marked *
Course Features





